Prof. Bogdan Gabrys
Bournemouth University, UKRobust adaptive predictive modeling and data deluge
Abstract:
We are currently experiencing an incredible, explosive growth in digital content and information. According to IDC [11], there currently exists over 2.7 zetabytes of data. It is estimated that the digital universe in 2020 will be 50 times as big as in 2010 and that from now until 2020 it will double every two years. Research in traditionally qualitative disciplines is fundamentally changing due to the availability of such vast amounts of data. In fact, data-intensive computing has been named as the fourth paradigm of scientific discovery [10] and is expected to be key in unifying the theoretical, experimental and simulation based approaches to science. The commercial world has also been transformed by a focus on BIG DATA with companies competing on analytics [12]. Data has become a commodity and in recent years has been referred to as the 'new oil'.
There has been a lot of work done on the subject of intelligent data analysis, data mining and predictive modelling over the last 50 years with notable improvements which have been possible with both the advancements of the computing equipment as well as with the improvement of the algorithms [1]. However, even in the case of the static, non-changing over time data there are still many hard challenges to be solved which are related to the massive amounts, high dimensionality, sparseness or inhomogeneous nature of the data to name just a few.
What is also very challenging in today's applications is the non-stationarity of the data which often change very quickly posing a set of new problems related to the need for robust adaptation and learning over time. In scenarios like these, many of the existing, often very powerful, methods are completely inadequate as they are simply not adaptive and require a lot of maintenance attention from highly skilled experts, in turn reducing their areas of applicability.
In order to address these challenging issues and following various inspirations coming from biology coupled with current engineering practices, we propose a major departure from the standard ways of building adaptive, intelligent predictive systems and moving somewhat away from the engineering maxim of "simple is beautiful" to biological statement of "complexity is not a problem" by utilising the biological metaphors of redundant but complementary pathways, interconnected cyclic processes, models that can be created as well as destroyed in easy way, batteries of sensors in form of pools of complementary approaches, hierarchical organisation of constantly optimised and adaptable components.
In order to achieve such high level of adaptability we have proposed a novel flexible architecture [5-6] which encapsulates many of the principles and strategies observed in adaptable biological systems. The main idea of the proposed architecture revolves around a certain degree of redundancy present at each level of processing represented by the pools of methods, multiple competitive paths (individual predictors), their flexible combinations and meta learning managing general population and ensuring both efficiency and accuracy of delivered solution while maintaining diversity for improved robustness of the overall system.
The results of extensive testing for many different benchmark problems and various snapshots of interesting results covering the last decade of our research will be shown throughout the presentation and a number of challenging real world problems including pollution/toxicity prediction studies [8-9], building adaptable soft sensors in process industry in collaboration with Evonik Industries [6-7] or forecasting demand for airline tickets covering the results of one of our collaborative research projects with Lufthansa Systems [3-4] will be discussed.
Given our experiences in many different areas we see that truly multidisciplinary teams and a new set of robust, adaptive tools are needed to tackle complex problems with intelligent data analysis, predictive modelling and visualisation already indispensible. It is also clear that complex adaptive systems and complexity science supported and driven by huge amounts of multimodal, multisource data will become a major endeavour in the 21st century.
References
- Gabrys, B., K. Leiviska and J. Strackeljan (Eds.): Do Smart Adaptive Systems Exist? - Best Practice for Selection and Combination of Intelligent Methods. Springer series on "Studies in Fuzziness and Soft Computing", 2005
- Ruta, D. and B. Gabrys, "Classifier Selection for Majority Voting", Information Fusion. Special Issue on Diversity in Multiple Classifier Systems, vol. 6, issue 1, pp. 63-81, 2005.
- Riedel, S. and B. Gabrys, "Combination of Multi Level Forecasts", International Journal of VLSI Signal Processing Systems. Special issue on "Data Fusion for Medical, Industrial, and Environmental Applications", vol. 49, no. 2, pp. 265-280, 2007.
- Riedel, S. and B. Gabrys, "Pooling for Combination of Multi Level Forecasts", IEEE Transactions on Knowledge and Data Engineering, 21 (12), pp. 1753-1766, Dec 2009.
- Ruta, D. and B. Gabrys and C. Lemke, "A Generic Multilevel Architecture for Time Series Prediction", IEEE Transactions on Knowledge and Data Engineering, In press. Dec 2009.
- Kadlec, P. and B. Gabrys, "Architecture for development of adaptive on-line prediction models", Memetic Computing, 1 (4), pp. 241-269. Dec. 2009.
- Kadlec, P., B. Gabrys and S. Strandt, "Data-driven Soft Sensors in the Process Industry", Computers and Chemical Engineering, 33 (4), pp. 795-814, 2009.
- Budka, M., Gabrys, B. and Ravagnan, E., "Robust predictive modelling of water pollution using biomarker data". Water Research. 44(10), pp. 3294-3308, May 2010.
- Budka, M. and Gabrys, B., "Ridge regression ensemble for toxicity prediction", Procedia Computer Science, 1(1), pp. 193-201, May 2010.
- Hey, T., S. Tansley and K. Tolle (EDs.): The Fourth Paradigm: Data-Intensive Scientific Discovery. Microsoft Press, 2009.
- Gantz, J., and D. Reinsel, "THE DIGITAL UNIVERSE IN 2020: Big Data, Bigger Digital Shadows, and Biggest Growth in the Far East", http://www.emc.com/collateral/analyst-reports/idc-the-digital-universe-in-2020.pdf, Sponsored by EMC. Dec. 2012
- Davenport, T.H., and J.G. Harris, Competing on Analytics: The New Science of Winning. Harvard Business School Press, 2007
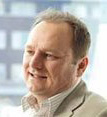
Biography:
After many years of working at different Universities, Prof Gabrys moved to the Bournemouth University in January 2003 where he acts as a Director of the Smart Technology Research Centre and a Head of the Computational Intelligence Research Group within the School of Design, Engineering & Computing. His current research interests lay in a broad area of intelligent and biologically/nature inspired learning and complex adaptive systems and include a wide range of machine learning and hybrid intelligent techniques encompassing data and information fusion, learning and adaptation methods, multiple classifier and prediction systems, complex adaptive systems, processing and modelling of uncertainty in predictive analytics, pattern recognition, diagnostic analysis and decision support systems.
Among others, Prof. Gabrys is currently co-ordinating an EU funded INFER project (http://www.infer.eu), acts as the Chair (Academic Affairs) of KES International and is a Co-Editor in Chief of the International Journal of Knowledge Based & Intelligent Engineering Systems.
He was a co-chairman of the Nature-inspired Data Technology focus group within an EU funded Coordination Action project on Nature-inspired Smart Information Systems (NiSIS). He was also a corresponding person for a Key Node in the European Network of Excellence on Intelligent Technologies for Smart Adaptive Systems (EUNITE) and a co-chairman of the Research Theory & Development Group on Integration of Methods.
More information can be found at his personal web page: http://dec.bournemouth.ac.uk/staff/bgabrys/Back to Keynote Speakers